Factus (high-level)
This section will walk you through using FactCast from an application programmers perspective using the abstractions of
Factus. Factus is an optional high-level API provided in order to make it easier to work with FactCast from Java (or
Kotlin or any other JVM language of your choice).
It has dedicated modules to integrate with several datastores for storage of snapshots and other projections. For
details have a look at Projections.
Please be aware that the Factus API is in experimental stage and is expected to change while getting more mature.
If you find sharp edges or feel like things are missing,
please open an issue on GitHub.
If you want to have more control and don’t want to opt in to Factus, you can have a look at the lower-level FactCast
API. See the Lowlevel section instead.
1 - Introduction
Motivation
If Factus is optional, why does it exist in the first place, you might ask.
FactCast tries to be non-intrusive. It focuses on publishing, retrieval, validation and transformation of JSON documents. It also provides some tools for advanced (yet necessary) concepts like optimistic locking, but it does not prescribe anything in terms of how to use this to build an application.
Depending on your experience with eventsourcing in general or other products/approaches in particular, it might be hard to see how exactly this helps you to build correct, scalable and maintainable systems. At least this was our experience working with diverse groups of engineers over the years.
Now, instead of documenting lots of good practices here, we thought it would be easier to start with, more convenient and less error-prone to offer a high-level API instead, that codifies those good practices.
We say “good” practices here, rather than “best” practices for a reason. Factus represents just one way of using FactCast from Java. Please be aware that it may grow over time and that there is nothing wrong with using a different approach.
Also, be aware that not every possible use case is covered by Factus so that you occasionally might want to fall back to “doing things yourself” with the low-level FactCast API.
In case you encounter such a situation, please open a GitHub issue explaining your motivation. Maybe this is something Factus is currently lacking.
Factus as a higher level of abstraction
Factus replaces FactCast as a central interface. Rather than with Facts, Factus primarily deals with EventObjects deserialized from Facts.
using an EventSerializer. Factus ships with a default one that uses Jackson, but you’re free to use any library of your taste to accomplish this (like Gson, or whatever is popular with you).
Concrete events will implement EventObject in order to be able to contribute to Fact Headers when serialized, and they are expected to be annotated with @Specification
in order to declare what the specifics of the FactHeader
(namespace, type and version) are.
import com.google.common.collect.Sets;
/**
* EventObjects are expected to be annotated with @{@link Specification}.
*/
public interface EventObject {
default Map<String, String> additionalFactHeaders() {
return Collections.emptyMap();
}
Set<UUID> aggregateIds();
}
/**
* Example EventObject based event containing one property
*/
@Specification(ns = "user", type = "UserCreated", version = 1)
class UserCreated implements EventObject {
// getters & setters or builders omitted
private UUID userId;
private String name;
@Override
public Set<UUID> aggregateIds() {
return Sets.newHashSet(userId);
}
}
Now the payload of a Fact created from your Events will be, as you’d expect, the json-serialized form of the Event which is created by the EventSerializer
.
Factus ships with a default serializer for EventObjects. It uses Jackson and builds on a predefined ObjectMapper, if defined (otherwise just uses the internal FactCast-configured ObjectMapper).
If, for some reason, you want to redefine this, you can use/ provide your own EventSerializer.
As factus is optional, you’ll first want to setup you project to use it. See Factus Setup
2 - Setup
Dependencies
First thing you need in your project is a dependency to factus itself.
<dependency>
<groupId>org.factcast</groupId>
<artifactId>factcast-factus</artifactId>
</dependency>
If you use Spring-Boot and also have the spring boot autoconfiguration dependency included,
<dependency>
<groupId>org.factcast</groupId>
<artifactId>factcast-spring-boot-autoconfigure</artifactId>
</dependency>
this is all you need to get started.
However, there is a growing list of optional helpful dependencies when it comes to using Factus:
Binary Snapshot Serializer
The default Snapshot Serializer in Factus uses Jackson to serialize to/from JSON. This might be less than optimal in terms of storage cost and transport performance/efficiency.
This optional dependency:
<dependency>
<groupId>org.factcast</groupId>
<artifactId>factcast-factus-bin-snapser</artifactId>
</dependency>
replaces the default Snapshot Serializer by another variant, that - while still using jackson to stay compatible
with the default one from the classes perspective - serializes to a binary format and uses lz4 to swiftly (de-)compress
it on the fly.
Depending on your environment, you may want to roll your own and use a slower, but more compact compression or maybe
just want to switch to plain Java Serialization. In this case, have a look at BinarySnapshotSerializer
to explore, how to do it.
(If you do, please contribute it back - might be worthwhile integrating into factcast)
Should be straightforward and easy.
In case you want to configure this serializer, define a BinaryJacksonSnapshotSerializerCustomizer
bean and
define the configuration in there. Take a look at BinaryJacksonSnapshotSerializerCustomizer#defaultCustomizer
if you need inspiration.
Redis SnapshotCache
From a client’s perspective, it is nice to be able to persist snapshots directly into factcast, so that you don’t
need any additional infrastructure to get started. In busy applications with many clients however, it might be
a good idea to keep that load away from factcast, so that it can use its capacity to deal with Facts only.
In this case you want to use a different implementation of the SnapshotCache interface on a client, in order to
persist snapshots in your favorite K/V store, Document Database, etc.
We chose Redis as an example database for externalized shared data for the examples, as it has a very simple API and is
far more lightweight to use than a RDBMS. But, please be aware, that you can use ANY Database to store shared data
and snapshots, by just implementing the respective interfaces.
In case Redis is you weapon of choice, there is a Redis implementation of that interface. Just add
<dependency>
<groupId>org.factcast</groupId>
<artifactId>factcast-snapshotcache-redisson</artifactId>
</dependency>
to your client’s project and spring autoconfiguration (if you use spring boot) will do the rest.
As it relies on the excellent Redisson library, all you need is to add the corresponding redis configuration to your project.
See the Redisson documentation.
3 - Publication
The publishing side is easy and should be intuitive to use. Factus offers a few methods to publish either Events (or Facts if you happen to have handcrafted ones) to FactCast.
public interface Factus extends SimplePublisher, ProjectionAccessor, Closeable {
/**
* publishes a single event immediately
*/
default void publish(@NonNull EventObject eventPojo) {
publish(eventPojo, f -> null);
}
/**
* publishes a list of events immediately in an atomic manner (all or none)
*/
default void publish(@NonNull List<EventObject> eventPojos) {
publish(eventPojos, f -> null);
}
/**
* publishes a single event immediately and transforms the resulting facts
* to a return type with the given resultFn
*/
<T> T publish(@NonNull EventObject e, @NonNull Function<Fact, T> resultFn);
/**
* publishes a list of events immediately in an atomic manner (all or none)
* and transforms the resulting facts to a return type with the given
* resultFn
*/
<T> T publish(@NonNull List<EventObject> e, @NonNull Function<List<Fact>, T> resultFn);
/**
* In case you'd need to assemble a fact yourself
*/
void publish(@NonNull Fact f);
// ...
As you can see, you can either call a void method, or pass a function that translates the published Facts to a return value, in case you need it.
Batches
Just like FactCast’s publish(List<Fact>)
, you can publish a list of Events/Facts atomically.
However, in some more complex scenarios, it might be more appropriate to have an object to pass around (and maybe mark aborted) where different parts of the code can contribute Events/Facts to publish to.
This is what PublishBatch is used for:
public interface PublishBatch extends AutoCloseable {
PublishBatch add(EventObject p);
PublishBatch add(Fact f);
void execute() throws BatchAbortedException;
<R> R execute(Function<List<Fact>, R> resultFunction) throws BatchAbortedException;
PublishBatch markAborted(String msg);
PublishBatch markAborted(Throwable cause);
void close(); // checks if either aborted or executed already, otherwise will execute
}
In order to use this, just call Factus::batch
to create a new PublishBatch object.
4 - Projection

Before we can look at processing Events, we first have to talk about another abstraction that does not exist in
FactCast: Projection
public interface Projection { ...
}
In Factus, a Projection is any kind of state that is distilled from processing Events - in other words: Projection
s
process (or handle) events.
Persistence / Datastores
Projections are meant to handle Events and create queryable models from them. While these Models can live anywhere (from
in-memory to your fancy homegrown database solution), there are a bunch of modules in the factcast project that make
integration with foreign datastores easier.
At the point of writing, there is (partly transactional) support for:
local projections
external projections
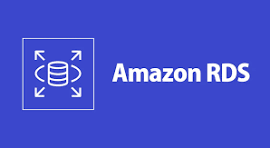
or any other via
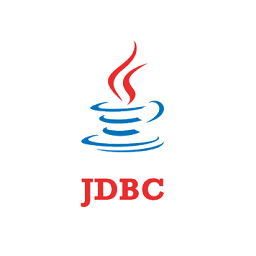
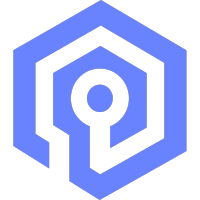

with more to come.
Projections in general
What projections have in common is that they handle Events (or Facts). In order to express that, a projection can have
any number of methods annotated with @Handler
or @HandlerFor
. These methods must be package-level/protected
accessible and can be either on the Projection itself or on a nested (non-static) inner class.
A simple example might be:
/**
* maintains a map of UserId->UserName
**/
public class UserNames implements SnapshotProjection {
private final Map<UUID, String> existingNames = new HashMap<>();
@Handler
void apply(UserCreated created) {
existingNames.put(created.aggregateId(), created.userName());
}
@Handler
void apply(UserDeleted deleted) {
existingNames.remove(deleted.aggregateId());
}
// ...
Here the EventObject ‘UserDeleted’ and ‘UserCreated’ are just basically tuples of a UserId (aggregateId) and a Name (
userName).
Also, projections must have a default (no-args) constructor.
As we established before, you could also decide to use a nested class to separate the methods from other instance
methods, like:
public class UserNames implements SnapshotProjection {
private final Map<UUID, String> existingNames = new HashMap<>();
class EventProcessing {
@Handler
void apply(UserCreated created) {
existingNames.put(created.aggregateId(), created.userName());
}
@Handler
void apply(UserDeleted deleted) {
existingNames.remove(deleted.aggregateId());
}
}
// ...
many Flavours
There are several kinds of Projections that we need to look at. But before, let’s start
with Snapshotting…
4.1 - Snapshotting
In EventSourcing a Snapshot is used to memorize an object’s state at a certain point in the EventStream, so that when later-on this object has to be retrieved again,
rather than creating a fresh one and use it to process all relevant events, we can start with the snapshot (that already has the state of the object from before)
and just process all the facts that happened since.
It is easy to see that storing and retrieving snapshots involves some kind of marshalling and unmarshalling, as well as some sort of Key/Value store to keep the snapshots.
Snapshot Serialization
Serialization is done using a SnapshotSerializer
.
public interface SnapshotSerializer {
byte[] serialize(SnapshotProjection a);
<A extends SnapshotProjection> A deserialize(Class<A> type, byte[] bytes);
/**
* @return displayable name of the serializer. Make sure it is unique, as it is used as part of
* the snapshot key
*/
SnapshotSerializerId id();
}
As you can see, there is no assumption whether it produces JSON or anything, it just has to be symmetric.
Factus ships with a default SnapshotSerializer, that - you can guess by now - uses Jackson. Neither the
most performant, nor the most compact choice. Feel free to create one on your own or use provided optional modules
like:
factcast-factus-serializer-binary
or factcast-factus-serializer-fury
Choosing serializers
If your SnapshotProjection
does not declare anything different, it will be serialized using the default SnapshotSerializer known to your SnapshotSerializerSupplier
(when using Spring boot, normally automatically bound as a Spring bean).
In case you want to use a different implementation for a particular ‘SnapshotProjection’, you can annotate it with ‘@SerializeUsing’
@SerializeUsing(MySpecialSnapshotSerializer.class)
static class MySnapshotProjection implements SnapshotProjection {
//...
}
Note that those implementations need to have a default constructor and are expected to be stateless.
However, if you use Spring boot those implementations can be Spring beans as well which are then retrieved from the Application Context via the type provided in the annotation.
Snapshot caching
The Key/Value store that keeps and maintains the snapshots is called a SnapshotCache.
Revisions
When a projection class is changed (e.g. a field is renamed or its type is changed), depending on the Serializer, there will be a problem with deserialization.
In order to rebuild a snapshot in this case a “revision” is to be provided for the Projection.
Only snapshots that have the same “revision” than the class in its current state will be used.
Revisions are declared to projections by adding a @ProjectionMetaData(revision = 1L)
to the type.
4.1.1 - Snapshot Caching
The component responsible for storing and managing snapshots is called the SnapshotCache.
Factus does not provide a default SnapshotCache, requiring users to make an explicit configuration choice. If a
SnapshotCache is not configured, any attempt to use snapshots will result in an UnsupportedOperationException.
By default, the SnapshotCache retains only the latest version of a particular snapshot.
There are several predefined SnapshotCache implementations available, with plans to introduce additional options in the
future.
In-Memory SnapshotCache
For scenarios where persistence and sharing of snapshots are not necessary, and sufficient RAM is available, the
in-memory solution can be used:
<dependency>
<groupId>org.factcast</groupId>
<artifactId>factcast-snapshotcache-local-memory</artifactId>
</dependency>
Refer to the In-Memory Properties for configuration details.
In-Memory and Disk SnapshotCache
To persist snapshots on disk, consider using the following configuration:
<dependency>
<groupId>org.factcast</groupId>
<artifactId>factcast-snapshotcache-local-disk</artifactId>
</dependency>
Note that this setup is designed for single-instance applications and handles file access synchronization within the
active instance. It is not recommended for distributed application architectures.
Refer to the In-Memory and Disk Properties for more
information.
Redis SnapshotCache
For applications utilizing Redis, the Redis-based SnapshotCache offers an optimal solution to implement a
snapshotcache that can be shared between / used by many instances:
<dependency>
<groupId>org.factcast</groupId>
<artifactId>factcast-snapshotcache-redisson</artifactId>
</dependency>
This option supports multiple instances of the same application, making it suitable for distributed environments. By
default, this cache automatically deletes stale snapshots after 90 days.
For further details, see the Redis Properties.
JDBC SnapshotCache
For applications utilizing a JDBC storage solution, the JDBC-based SnapshotCache offers an optimal solution:
<dependency>
<groupId>org.factcast</groupId>
<artifactId>factcast-snapshotcache-jdbc</artifactId>
</dependency>
This option also enables the use of multiple instances of the same application, facilitating distributed environments by
leveraging the ACID properties of the databases. Additionally, the cache is configured by default to automatically
purge stale snapshots after 90 days.
⚠️ Warning: This SnapshotCache assumes that you already created the needed table with the specified schema and
will fail on startup when this condition is not met.
You can run one of the following SQL scripts to create the necessary table:
PostgreSQL
CREATE TABLE IF NOT EXISTS factcast_snapshot(
projection_class VARCHAR(512),
aggregate_id VARCHAR(36) NULL,
last_fact_id VARCHAR(36),
bytes BYTEA,
snapshot_serializer_id VARCHAR(128),
last_accessed VARCHAR,
PRIMARY KEY (projection_class, aggregate_id));
CREATE INDEX IF NOT EXISTS factcast_snapshot_last_accessed_index ON factcast_snapshot(last_accessed);
MySQL & MariaDB
CREATE TABLE IF NOT EXISTS factcast_snapshot (
projection_class VARCHAR(512) NOT NULL,
aggregate_id VARCHAR(36) NULL,
last_fact_id VARCHAR(36) NOT NULL,
bytes BLOB,
snapshot_serializer_id VARCHAR(128) NOT NULL,
last_accessed VARCHAR(255),
PRIMARY KEY (projection_class, aggregate_id)
);
CREATE INDEX factcast_snapshot_last_accessed_index ON factcast_snapshot (last_accessed);
Oracle
CREATE TABLE factcast_snapshot (
projection_class VARCHAR2(512) NOT NULL,
aggregate_id VARCHAR2(36) NULL,
last_fact_id VARCHAR2(36) NOT NULL,
bytes BLOB,
snapshot_serializer_id VARCHAR2(128) NOT NULL,
last_accessed VARCHAR2(255),
PRIMARY KEY (projection_class, aggregate_id)
);
CREATE INDEX factcast_snapshot_last_accessed_index ON factcast_snapshot (last_accessed);
For further details, see the JDBC Properties.
4.2 - Projection Types

Use the Menu on the left hand side to learn about the different flavors of projections.
4.2.1 - Snapshot

Now that we know how snapshotting works and what a projection is, it is quite easy to put things together:
A SnapshotProjection is a Projection (read EventHandler) that can be stored into/created from a Snapshot. Let’s go back
to the example we had before:
/**
* maintains a map of UserId->UserName
**/
public class UserNames implements SnapshotProjection {
private final Map<UUID, String> existingNames = new HashMap<>();
@Handler
void apply(UserCreated created) {
existingNames.put(created.aggregateId(), created.userName());
}
@Handler
void apply(UserDeleted deleted) {
existingNames.remove(deleted.aggregateId());
}
// ...
This projection is interested in UserCreated
and UserDeleted
EventObjects and can be serialized by
the SnapshotSerializer
.
If you have worked with FactCast before, you’ll know what needs to be done (if you haven’t, just skip this section and
be happy not to be bothered by this anymore):
- create an instance of the projection class, or get a Snapshot from somewhere
- create a list of FactSpecs (FactSpecifications) including the Specifications from
UserCreated
and UserDeleted
- create a FactObserver that implements an
void onNext(Fact fact)
method, that- looks at the fact’s namespace/type/version
- deserializes the payload of the fact into the right EventObject’s instance
- calls a method to actually process that EventObject
- keeps track of facts being successfully processed
- subscribe to a fact stream according to the FactSpecs from above (either from Scratch or from the last factId
processed by the instance from the snapshot)
- await the completion of the subscription to be sure to receive all EventObjects currently in the EventLog
- maybe create a snapshot manually and store it somewhere, so that you do not have to start from scratch next time
… and this is just the “happy-path”.
With Factus however, all you need to do is to use the following method:
/**
* If there is a matching snapshot already, it is deserialized and the
* matching events, which are not yet applied, will be as well. Afterwards, a new
* snapshot is created and stored.
* <p>
* If there is no existing snapshot yet, or they are not matching (see
* serialVersionUID), an initial one will be created.
*
* @return an instance of the projectionClass in at least initial state, and
* (if there are any) with all currently published facts applied.
*/
@NonNull
<P extends SnapshotProjection> P fetch(@NonNull Class<P> projectionClass);
like
UserNames currentUserNames=factus.fetch(UserNames.class);
Easy, uh? As the instance is created from either a Snapshot or the class, the instance is private to the caller here.
This is the reason why there is no ConcurrentHashMap or any other kind of synchronization necessary within UserNames
.
Lifecycle hooks
There are plenty of methods that you can override in order to hook into the lifecycle of a SnapshotProjection.
- onCatchup() - will be called when the catchup signal is received from the server.
- onComplete() - will be called when the FactStream is at its end (only valid for catchup projections)
- onError() - whenever an error occurs on the server side or on the client side before applying a fact
- onBeforeSnapshot() - will be called whenever factus is about to take a snapshot of the projection. Might be an
opportunity to clean up.
- onAfterRestore() - will be called whenever factus deserializes a projection from a snapshot. Might be an opportunity
to initialize things.
- executeUpdate(Runnable) - will be called to update the state of a projection. The runnable includes applying the Fact/Event and also updating the state of the projection, in case you want to do something like introduce transactionality here.
This is not meant to be an exhaustive list. Look at the interfaces/classes you implement/extend and their javadoc.
4.2.2 - Aggregate

Another special flavor of a Snapshot Projection is an Aggregate. An Aggregate extends the notion on Snapshot Projection by bringing in an aggregate Id. This is the one of the UserNames
example. It does not make sense to maintain two different UserNames Projections, because by definition, the UserNames projection should contain all UserNames in the system.
When you think of User
however, you have different users in the System that differ in Id and (probably) UserName.
So calling factus.fetch(User.class)
would not make any sense. Here Factus offers two different methods for access:
/**
* Same as fetching on a snapshot projection, but limited to one
* aggregateId. If no fact was found, Optional.empty will be returned
*/
@NonNull
<A extends Aggregate> Optional<A> find(
@NonNull Class<A> aggregateClass,
@NonNull UUID aggregateId);
/**
* shortcut to find, but returns the aggregate unwrapped. throws
* {@link IllegalStateException} if the aggregate does not exist yet.
*/
@NonNull
default <A extends Aggregate> A fetch(
@NonNull Class<A> aggregateClass,
@NonNull UUID aggregateId) {
return find(aggregateClass, aggregateId)
.orElseThrow(() -> new IllegalStateException("Aggregate of type " + aggregateClass
.getSimpleName() + " for id " + aggregateId + " does not exist."));
}
As you can see, find
returns the user as an Optional (being empty if there never was any EventObject published regarding that User), whereas fetch
returns the User unwrapped and fails if there is no Fact for that user found.
All the rules from SnapshotProjections apply: The User instance is (together with its id) stored as a snapshot at the end of the operation. You also have the beforeSnapshot() and afterRestore() in case you want to hook into the lifecycle (see SnapshotProjection)
4.2.3 - Managed

As we have learnt, SnapshotProjections are created from scratch or from Snapshots, whenever you fetch them.
If you look at it from another angle, you could call them unmanaged in a sense, that the application has no
control over their lifecycle.
There are use cases where this is less attractive. Consider a query model that powers a high-traffic REST API.
Recreating an instance of a SnapshotProjection for every query might be too much of an overhead caused by the
network transfer of the snapshot and the deserialization involved.
Considering this kind of use, it would be good if the lifecycle of the Model would be managed by the application.
It also means, there must be a way to ‘update’ the model when needed (technically, to process all the Facts that have
not yet been applied to the projection).
However, if the Projection is application managed (so that it can be shared between threads) but needs to be updated
by catching up with the Fact-Stream, there is a problem we did not have with SnapshotProjections, which is
locking.
Definition
A ManagedProjection is a projection that is managed by the Application. Factus can be used to lock/update/release
a Managed Projection in order to make sure it processes Facts in the correct order and uniquely.
Factus needs to make sure only one thread will change the Projection by catching up with the latest Facts.
Also, when Factus has no control over the Projection, the Projection implementation itself needs to ensure
that proper concurrency handling is implemented in the place the Projection is being queried from, while being updated.
Depending on the implementation strategy used by you, this might be something you don’t need to worry about (for
instance when using a transactional datastore).
ManagedProjections are StateAware
(they know their position in the FactStream) and WriterTokenAware
, so that
they provide a way for Factus to coordinate updates.
flexible update
One of the most important qualities of ManagedProjections is that they can be updated at any point.
This makes them viable candidates for a variety of use cases. A default one certainly is a “strictly consistent”
model, which can be used to provide consistent reads over different nodes that always show the latest state from
the fact stream. In order to achieve this, you’d just update the model before reading from it.
// let's consider userCount is a spring-bean
UserCount userCount = new UserCount();
// now catchup with the published events
factus.update(userCount);
Obviously, this makes the application dependent on the event store for availability (and possibly latency).
The good part however is, that if FactCast was unavailable, you’d still have (a potentially) stale model you can
fall back to.
In cases where consistency with the fact-stream is not that important, you might just want to occasionally update
the model. An example would be to call update for logged-in users (to make sure, they see their potential writes)
but not updating for public users, as they don’t need to see the very latest changes.
One way to manage the extends of “staleness” of a ManagedProjection could be just a scheduled update call,
once every 5 minutes or whatever your requirements are for public users.
private final UserCount userCount;
private final Factus factus;
@Scheduled(cron = "*/5 * * * *")
public void updateUserCountRegularly(){
factus.update(userCount);
}
If the projection is externalized and shared, keep in mind that your users still get a consistent view of the system,
because all nodes share the same state.
Typical implementations
ManagedProjections are often used where the state of the projection is externalized and potentially shared between
nodes. Think of JPA Repositories or a Redis database.
The ManagedProjection instance in the application should provide access to the externalized data and implement
the locking facility.
Over time, there will be some examples added here with exemplary implementations using different technologies.
However, ManagedProjections do not have to work with externalized state. Depending on the size of the
Projection and consistency requirements between nodes, it might also be a good idea to just have an in-process (local)
representation of the state. That makes at least locking much easier.
Let’s move on to LocalManagedProjections…
4.2.4 - Managed (local)

As a specialization of ManagedProjection, a LocalManagedProjection lives within the application
process and does not use shared external Databases to maintain its state.
Relying on the locality, locking and keeping track of the state (position in the eventstream) is
just a matter of synchronization and an additional field, all being implemented in the abstract
class LocalManagedProjection
that you are expected to extend.
public class UserCount extends LocalManagedProjection {
private int users = 0;
@Handler
void apply(UserCreated created) {
users++;
}
@Handler
void apply(UserDeleted deleted) {
users--;
}
int count() {
return users;
}
}
As you can see, the WriterTokenBusiness and the state management are taken care of for you, so that you can just
focus on the implementation of the projection.
Due to the simplicity of use, this kind of implementation would be attractive for starting
with for non-aggregates, assuming the data held by the Projection is not huge.
4.2.5 - Subscribed

The SnapshotProjection
and ManagedProjection
have one thing in common:
The application actively controls the frequency and time of updates by actively calling a method. While this gives the
user a maximum of control, it also requires synchronicity. Especially when building query models, this is not
necessarily a good thing. This is where the SubscribedProjection
comes into play.
Definition
A SubscribedProjection
is subscribed once to a Fact-stream and is asynchronously updated as soon as the application
receives relevant facts.
Subscribed projections are created by the application and subscribed (once) to factus. As soon as Factus receives
matching Facts from the FactCast Server, it updates the projection. The expected latency is obviously dependent on a
variety of parameters, but under normal circumstances it is expected to be <100ms, sometimes <10ms.
However, its strength (being updated in the background) is also its weakness: the application never knows what state the
projection is in (eventual consistency).
While this is a perfect projection type for occasionally connected operations or public query models, the inherent
eventual consistency might be confusing to users, for instance in a read-after-write scenario, where the user
does not see his own write. This can lead to suboptimal UX und thus should be used cautiously after carefully
considering the trade-offs.
A SubscribedProjection
is also StateAware
and WriterTokenAware
. However, the token will not be released as frequently
as with a ManagedProjection
. This may lead to “starving” models, if the process keeping the lock is non-responsive.
Please keep that in mind when implementing the locking facility.
Read-After-Write Consistency
Factus updates subscribed projections automatically in the background. Therefore a manual update with
``
factus.update(projection)
is not possible. In some cases however it might still be necessary to make sure a subscribed
projection has processed a fact before continuing.
One such use-case might be read-after-write consistency. Imagine a projection powering a table shown to a user. This
table shows information collected from facts A
and B
, where B
gets published by the current application, but
A
is published by another service, which means we need to use a subscribed projection. With the push of a button a user can publish a new B
fact, creating another row
in the table. If your frontend then immediately reloads the table, it might not yet show the new row, as the subscribed
projection has not yet processed the new fact.
In this case you can use the factus.waitFor
method to wait until the projection has consumed a certain fact. This
method will block until the fact is either processed or the timeout is exceeded.
// publish a fact we need to wait on and extract its ID
final var factId = factus.publish(new BFact(), Fact::id);
factus.waitFor(subscribedProjection, factId, Duration.ofSeconds(5));
With this, the waiting thread will block for up to 5 seconds or until the projection has processed the fact stream up to or beyond the specified fact.
If you use this, make sure that the projection you are waiting for will actually process the fact you are waiting on.
Otherwise a timeout is basically guaranteed, as the fact will never be processed by this projection.
4.2.6 - Subscribed (local)

As a specialization of the SubscribedProjection
, a LocalSubscribedProjection
is local to one VM (just like a LocalManagedProjection
).
This leads to the same problem already discussed in relation to LocalManagedProjection
: A possible inconsistency between nodes.
A LocalSubscribedProjection
is providing locking (trivial) and state awareness, so it is very easy to use/extend.
4.3 - Atomicity
Note
Atomic projections only make sense in terms of:
as snapshot projections (including Aggregates) work with local state & externalized snapshots and thus are atomic by design.
Introduction
When processing events, an externalized projection has two tasks:
- persist the changes resulting from the Fact
- store the current fact-stream-position
When using an external datastore (e.g. Redis, JDBC, MongoDB), Factus needs to ensure that these two tasks happen atomically: either both
tasks are executed or none. This prevents corrupted data in case e.g. the Datastore goes down in the wrong moment.
Factus offers atomic writes through atomic projections.
sequenceDiagram
participant Projection
participant External Data Store
Projection->>External Data Store: 1) update projection
Note right of External Data Store: Inside Transaction
Projection->>External Data Store: 2) store fact-stream-position
In an atomic Projection, the projection update and the update of the fact-stream-position need to run atomically
Factus currently supports atomicity for the following external data stores:
Note
There is an internal API available you can use to support your favorite data store.Configuration
Atomic projections are declared via specific annotations. Currently, supported are
These annotations share a common configuration attribute:
Parameter Name | Description | Default Value |
---|
bulkSize | how many events are processed in a bulk | 50 |
as well as, different attributes needed to configure the respective underlying technical solution (Transaction/Batch/…).
There are reasonable defaults for all of those attributes present.
Optimization: Bulk Processing
In order to improve the throughput of event processing, atomic projections support bulk processing.
With bulk processing
- the concrete underlying transaction mechanism (e.g. Spring Transaction Management) can optimize accordingly.
- skipping unnecessary fact-stream-position updates is possible (see next section).
The size of the bulk can be configured via a common bulkSize
attribute of
the @SpringTransactional
or @RedisTransactional
annotation.
Once the bulkSize is reached, or a configured timeout is triggered, the recorded operations of this bulk will be flushed to the datastore.
Skipping fact-stream-position Updates
Skipping unnecessary updates of the fact-stream-position reduces the writes to the external datastore,
thus improving event-processing throughput.
The concept is best explained with an example: Suppose we have three events which are processed by a transactional projection and the bulk size set to “1”.
Then, we see the following writes going to the external datastore:
sequenceDiagram
participant Projection
participant External Data Store
Projection->>External Data Store: event 1: update projection data
Projection->>External Data Store: event 1: store fact-stream-position
Projection->>External Data Store: event 2: update projection data
Projection->>External Data Store: event 2: store fact-stream-position
Projection->>External Data Store: event 3: update projection data
Projection->>External Data Store: event 3: store fact-stream-position
Processing three events with bulk size “1” - each fact-stream-position is written
As initially explained, here, each update of the projection is accompanied by an update of the fact-stream-position.
In order to minimize the writes to the necessary, we now increase the bulk size to “3”:
sequenceDiagram
participant Projection
participant External Data Store
Projection->>External Data Store: event 1: update projection data
Projection->>External Data Store: event 2: update projection data
Projection->>External Data Store: event 3: update projection data
Projection->>External Data Store: event 3: store fact-stream-position
Processing three events with bulk size “3” - only the last fact-stream-position written
This configuration change eliminates two unnecessary intermediate fact-stream-position updates.
The bulk is still executed atomically, so in terms of fact-stream-position updates, we are just interested
in the last, most recent position.
Skipping unnecessary intermediate updates to the fact-stream-position, noticeably reduces
the required writes to the external datastore. Provided a large enough bulk size (“50” is a reasonable default),
this significantly improves event-processing throughput.
Note
‘Large enough’ of course depends on multiple factors like network, storage, etc.
Your mileage may vary.4.3.1 - Spring Transactional
Broad Data-Store Support
Spring comes with extensive support for transactions
which is employed by Spring Transactional Projections.
Standing on the shoulders of Spring Transactions,
Factus supports transactionality for every data-store for which Spring transaction management
is available. In more detail, for the data-store in question, an implementation of the Spring PlatformTransactionManager
must exist.
Motivation
You would want to use Spring Transactional for two reasons:
- atomicity of factStreamPosition updates and your projection state updates
- increased fact processing throughput
The Performance bit is achieved by skipping unnecessary factStreamPosition updates and (more importantly) by reducing the number of transactions on your datastore by using one Transaction for bulkSize
updates instead of single writes.
For instance, if you use Spring Transactions on a JDBC Datastore, you will have one database transaction around the update of bulkSize
events.
The bulkSize
is configurable per projection via the @SpringTransactional annotation.
Configuration
In order to make use of spring transaction support, the necessary dependency has to be included in your project:
<dependency>
<groupId>org.factcast</groupId>
<artifactId>factcast-factus-spring-tx</artifactId>
</dependency>
Structure
To use Spring Transactionality, a projection needs to:
- be annotated with
@SpringTransactional
to configure bulk and transaction-behavior and - implement
SpringTxProjection
to return the responsible PlatformTransactionManager for this kind of Projection
Applying facts
In your @Handler methods, you need to make sure you use the Spring-Managed Transaction when talking to your datastore.
This might be entirely transparent for you (for instance, when using JDBC that assigns the transaction to the current thread), or will need you to resolve the current transaction from the given platformTransactionManager
example.
Please consult the Spring docs or your driver’s documentation.
Note
Factus provides convenient abstract classes for managed and subscribed projections:
AbstractSpringTxManagedProjection
AbstractSpringTxSubscribedProjection
You can find blueprints of getting started in the example section.
4.3.2 - Redis Transactional
A Redis transactional projection is a transactional projection
based on Redisson RTransaction.
Compared to a Spring transactional projection, a Redis transactional projection is more lightweight since
- transactionality is directly provided by
RTransaction
. There is no need to deal with Spring’s PlatformTransactionManager
- the fact stream position is automatically managed (see example below)
Motivation
You would want to use Redis Transactional for two reasons:
- atomicity of factStreamPosition updates and your projection state updates
- increased fact processing throughput
The performance bit is achieved by skipping unnecessary factStreamPosition updates and (more importantly) by
reducing the number of operations on your Redis backend by using bulkSize
updates with one redisson transsaction
instead of single writes.
The bulkSize
is configurable per projection via the @RedisTransactional
annotation.
Working with a Redis transactional projection you can read your own uncommitted write. For this reason, a Redis transactional projection is best used for projections which
need to access the projection’s data during the handling of an event.
Configuration
In order to make use of redisson RTransaction support, the necessary dependency has to be included in your project:
<dependency>
<groupId>org.factcast</groupId>
<artifactId>factcast-factus-redis</artifactId>
</dependency>
Structure
A Redis transactional projection can be a managed- or
a subscribed projection and is defined as follows:
- it is annotated with
@RedisTransactional
(optional when using the default values and extending one of Factus’ abstract classes mentioned below) - it implements
RedisProjection
revealing the RedisClient
used - it provides the revision number of the projection via the
@ProjectionMetaData
annotation - the handler methods receive an additional
RTransaction
parameter
Note
Factus provides convenient abstract classes for managed and subscribed projections:
AbstractRedisTxManagedProjection
AbstractRedisTxSubscribedProjection
Example
@Handler
void apply(SomethingHappened fact, RTransaction tx) {
myMap = tx.getMap( ... ).put( fact.getKey() , fact.getValue() );
}
a full example can be found here
4.4 - Examples
In here, you will find some examples that you can use as a simple blueprint to get started building projections.
We make use of some abstract classes here that might be more convenient to use. Feel free to study the
implementations of those abstracts to see what is going on, especially when you plan to implement projections with
different datastore than what we use in the examples.
4.4.1 - UserNames (Spring/JDBC)
Here is an example for a managed projection externalizing its state to a relational database (PostgreSQL here) using Spring transactional management.
The example projects a list of used UserNames in the System.
Preparation
We need to store two things in our JDBC Datastore:
- the actual list of UserNames, and
- the fact-stream-position of your projection.
Therefore we create the necessary tables (probably using liquibase/flyway or similar tooling of your choice):
CREATE TABLE users (
name TEXT,
id UUID,
PRIMARY KEY (id));
CREATE TABLE fact_stream_positions (
projection_name TEXT,
fact_stream_position UUID,
PRIMARY KEY (projection_name));
Given a unique projection name, we can use fact_stream_positions as a common table for all our JDBC managed projections.
TODO
provide example for acquireWriteToken based on JDBCConstructing
Since we decided to use a managed projection, we extended the AbstractSpringTxManagedProjection
class.
To configure transaction management, our managed projection exposes the injected transaction manager to the rest of Factus by calling the parent constructor.
@ProjectionMetaData(serial = 1)
@SpringTransactional
public class UserNames extends AbstractSpringTxManagedProjection {
private final JdbcTemplate jdbcTemplate;
public UserNames(
@NonNull PlatformTransactionManager platformTransactionManager, JdbcTemplate jdbcTemplate) {
super(platformTransactionManager);
this.jdbcTemplate = jdbcTemplate;
}
...
As we’re making use of Spring here, we inject a PlatformTransactionManager
and a JdbcTemplate
here in order to communicate with the database in a transactional way.
Two remarks:
- As soon as your project uses the
spring-boot-starter-jdbc
dependency,
Spring Boot will automatically provide
you with a JDBC-aware PlatformTransactionManager. - To ensure that the database communication participates in the managed transaction,
the database access mechanism must be also provided by Spring. Thus, we suggest using the
JdbcTemplate
.
Configuration
The @SpringTransactional
annotation provides various configuration options:
Parameter Name | Description | Default Value |
---|
bulkSize | bulk size | 50 |
timeoutInSeconds | timeout in seconds | 30 |
Updating the projection
The two possible abstract base classes, AbstractSpringTxManagedProjection
or AbstractSpringTxSubscribedProjection
,
both require the following methods to be implemented:
Method Signature | Description |
---|
public UUID factStreamPosition() | read the last position in the Fact stream from the database |
public void factStreamPosition(@NonNull UUID factStreamPosition) | write the current position of the Fact stream to the database |
public WriterToken acquireWriteToken(@NonNull Duration maxWait) | coordinates write access to the projection, see here for details |
The first two methods tell Factus how to read and write the Fact stream’s position from the database.
Writing the fact position
Provided the table fact_stream_positions
exists, here is an example of how to write the Fact position:
@Override
public void factStreamPosition(@NonNull UUID factStreamPosition) {
jdbcTemplate.update(
"INSERT INTO fact_stream_positions (projection_name, fact_stream_position) " +
"VALUES (?, ?) " +
"ON CONFLICT (projection_name) DO UPDATE SET fact_stream_position = ?",
getScopedName().asString(),
factStreamPosition,
factStreamPosition);
}
For convenience, an UPSERT statement (Postgres syntax) is used, which INSERTs the UUID the first time
and subsequently only UPDATEs the value.
To avoid hard-coding a unique name for the projection, the provided method getScopedName()
is employed.
The default implementation makes sure the name is unique and includes the serial of the projection.
Reading the fact position
To read the last Fact stream position, we simply select the previously written value:
@Override
public UUID factStreamPosition() {
try {
return jdbcTemplate.queryForObject(
"SELECT fact_stream_position FROM fact_stream_positions WHERE projection_name = ?",
UUID.class,
getScopedName().asString());
} catch (IncorrectResultSizeDataAccessException e) {
// no position yet, just return null
return null;
}
}
In case no previous Fact position exists, null
is returned.
Applying Facts
When processing the UserCreated event, we add a new row to the users
tables, filled with event data:
@Handler
void apply(UserCreated e) {
jdbcTemplate.update(
"INSERT INTO users (name, id) VALUES (?,?);",
e.getUserName(),
e.getAggregateId());
}
When handling the UserDeleted event we do the opposite and remove the appropriate row:
@Handler
void apply(UserDeleted e) {
jdbcTemplate.update("DELETE FROM users where id = ?", e.getAggregateId());
}
We have finished the implementation of the event-processing part of our projection. What is missing is a way to
make the projection’s data accessible for users.
Querying the projection
Users of our projections (meaning “other code”) contact the projection via it’s public API.
Currently, there is no public method offering “user names”. So let’s change that:
public List<String> getUserNames() {
return jdbcTemplate.query("SELECT name FROM users", (rs, rowNum) -> rs.getString(1));
}
Using The Projection
Calling code that wants to talk to the projection, now just needs to call the getUserNames
method:
// create a local instance or get a Spring Bean from the ApplicationContext, depending on your code organization
UserNames userNameProjection = new UserNames(platformTransactionManager, jdbcTemplate);
// depending on many factors you *may* want to update the projection before querying it
factus.update(userNameProjection);
List<String> userNames = userNameProjection.getUserNames();
First, we create an instance of the projection and provide it with all required dependencies. As an alternative, you may want to let Spring manage the lifecycle of the projection
and let the dependency injection mechanism provide you an instance.
Next, we call update(...)
on the projection to fetch the latest events from the Fact stream. Note that when you use a pre-existing (maybe Spring managed singleton) instance of the projection, this step is optional and depends on your use-case. As last step, we ask
the projection to provide us with user names by calling getUserNames()
.
Full Example
To study the full example see
4.4.2 - UserNames (Redis Transactional)
Here is a projection that handles UserCreated and
UserDeleted events. It solves the same problem as the example we’ve seen in Spring transactional projections. However, this time we use Redis as our data store and Redisson as the access API.
Configuration
The @RedisTransactional
annotation provides various configuration options:
Parameter Name | Description | Default Value |
---|
bulkSize | bulk size | 50 |
timeout | timeout in milliseconds until a transaction is interrupted and rolled back | 30000 |
responseTimeout | timeout in milliseconds for Redis response. Starts to countdown when transaction has been successfully submitted | 5000 |
retryAttempts | maximum attempts to send transaction | 5 |
retryInterval | time interval in milliseconds between retry attempts | 3000 |
Constructing
Since we decided to use a managed projection, we extended the AbstractRedisTxManagedProjection
class.
To configure the connection to Redis via Redisson, we injected RedissonClient
in the constructor, calling the parent constructor.
@ProjectionMetaData(revision = 1)
@RedisTransactional
public class UserNames extends AbstractRedisTxManagedProjection {
public UserNames(RedissonClient redisson) {
super(redisson);
}
...
FactStreamPosition and Lock-Management are automatically taken care of by the underlying AbstractRedisManagedProjection
.
In contrast to non-atomic projections, when applying Facts to the Redis data structure, the instance variable userNames
cannot be used
as this would violate the transactional semantics. Instead, accessing and updating the
state is carried out on a transaction derived data-structure (Map
here) inside the handler methods.
Updating the projection
Applying Events
Received events are processed inside the methods annotated with @Handler
(the handler methods). To participate in
the transaction, these methods have an additional RTransaction
parameter which represents the current transaction.
Let’s have a closer look at the handler for the UserCreated
event:
@Handler
void apply(UserCreated e, RTransaction tx){
Map<UUID, String> userNames=tx.getMap(getRedisKey());
userNames.put(e.getAggregateId(),e.getUserName());
}
Note
RTransaction handling is the responsibility of Factus. As developers, you must not call e.g. commit()
or rollback()
yourself.In the previous example, the method getRedisKeys()
was used to retrieve the Redis key of the projection. Let’s have a
closer look at this method in the next section.
Default redisKey
The data structures provided by Redisson all require a unique identifier which is used to store them in Redis. The method getRedisKey()
provides an
automatically generated name, assembled from the class name of the projection and the serial number configured with
the @ProjectionMetaData
.
Additionally, an AbstractRedisManagedProjection
or a AbstractRedisSubscribedProjection
, as well as their transactional
(Tx
) counterparts, maintain the following keys in Redis:
getRedisKey() + "_state_tracking"
- contains the UUID of the last position of the Fact streamgetRedisKey() + "_lock"
- shared lock that needs to be acquired to update the projection.
Redisson API Datastructures vs. Java Collections
As seen in the above example, some Redisson data structures also implement the appropriate Java Collections interface.
For example, you can assign
a Redisson RMap
also to a standard Java Map
:
// 1) use specific Redisson type
RMap<UUID, String> = tx.getMap(getRedisKey());
// 2) use Java Collections type
Map<UUID, String> = tx.getMap(getRedisKey());
There are good reasons for either variant, 1)
and 2)
:
Full Example
@ProjectionMetaData(revision = 1)
@RedisTransactional
public class UserNames extends AbstractRedisTxManagedProjection {
private final Map<UUID, String> userNames;
public UserNames(RedissonClient redisson) {
super(redisson);
userNames = redisson.getMap(getRedisKey());
}
public List<String> getUserNames() {
return new ArrayList<>(userNames.values());
}
@Handler
void apply(UserCreated e, RTransaction tx) {
tx.getMap(getRedisKey()).put(e.getAggregateId(), e.getUserName());
}
@Handler
void apply(UserDeleted e, RTransaction tx) {
tx.getMap(getRedisKey()).remove(e.getAggregateId());
}
}
To study the full example, see
4.5 - Callbacks
When implementing the Projection interface, the user can choose to override these default hook methods for more fine-grained control:
Method Signature | Description |
---|
List<FactSpec> postprocess(List<FactSpec> specsAsDiscovered) | further filter the handled facts via their fact specification including aggregate ID and meta entries |
void onCatchup() | invoked after all past facts of the streams were processed. This is a good point to signal that the projection is ready to serve data (e.g. via a health indicator). |
void onComplete() | called when subscription closed without error |
void onError(Throwable exception) | called when subscription closed after receiving an error. The default impl is to simply logs the error. |
postprocess
Annotating your handler methods gives you a convenient way of declaring a projection’s interest into particular facts, filtered by ns
,type
,pojo to deserialize into, version etc.
This kind of filtering should be sufficient for most of the use-cases. However, annotations have to have constant attributes, so what you cannot do this way is to filter on values that are only available at runtime:
A particular aggregateId or a calculated meta-attribute in the header.
For these use-cases the postprocess hook can be used.
The following projection handles SomethingStarted
and SomethingEnded
events. When updating the projection, Factus invokes
the postprocess(...)
method and provides it with a list of FactSpec
specifications as discovered from the annotations.
If you override the default behavior here (which is just returning the list unchanged), you can intercept and freely modify, add or remove the FactSpecs
.
In our example this list will contain two entries with the FactSpec
built from the SomethingStarted
and ‘SomethingEnded’ classes respectively.
In the example only facts with a specific aggregate ID and the matching meta entry will be considered,
by adding these filters to every discovered FactSpec
.
public class MyProjection extends LocalManagedProjection {
@Handler
void apply(SomethingStarted event) { // ...
}
@Handler
void apply(SomethingEnded event) { // ...
}
@Override
public @NonNull List<FactSpec> postprocess(@NonNull List<FactSpec> specsAsDiscovered) {
specsAsDiscovered.forEach(
spec ->
// method calls can be chained
spec.aggId(someAggregateUuid)
.meta("someMetaAttribute", "someValue"));
return specsAsDiscovered;
}
Remember that filtering of facts via FactSpecs
takes place on the factcast server side.
onCatchup
The Factus API will call the onCatchup
method after an onCatchup signal was received from the server, indicating that the fact stream is now as near as possible to the end of the FactStream that is defined by the FactSpecs
used to filter.
Depending on the type of projection, the subscription now went from catchup
to follow
mode (for follow subscriptions), or is completed right after (for catchup subscriptions, see onComplete
).
One popular use-case for implementing the onCatchup
method is to signal the rest
of the service that the projection is ready to be queried and serve (not too stale) data.
In Spring for instance, a custom health indicator
can be used for that purpose.
@Override
public void onCatchup() {
log.debug("Projection is ready now");
// perform further actions e.g. switch health indicator to "up"
}
onComplete
The onComplete
method is called when the server terminated a subscription without any error. It is the last signal a server sends. The default behavior is to ignore this.
onError
The onError
method is called when the server terminated a subscription due to an error, or when one of your apply methods threw an exception. The subscription will be closed, either way.
The default behavior is to just log the error.
4.6 - Filtering
When implementing a Projection, you would add handler methods
(methods annotated with either @Handler
or @HandlerFor
)
in order to express, what the projection is interested in.
Factus will look at these methods in order to discover fact specifications.
These fact specifications form a query which is sent to the FactCast server to create a fact-stream suited for this projection.
In detail, for each handler method, a Projector inspects the method’s annotations and parameter types
including their annotations to build a
FactSpec
object.
This object contains at least the ns
, type
properties. Optionally the version
property is set.
If you look at a FactSpec
however, sometimes it makes sense to use additional filtering possibilities like
- aggregateId
- meta key/value pair (one or more) or even
- JavaScript acting as a predicate.
If for a projection these filters are known in advance, you can use additional annotations to declare them:
@FilterByAggId
@FilterByScript
@FilterByMeta
(can be used repeatedly)@FilterByMetaExists
(can be used repeatedly)@FilterByMetaDoesNotExist
(can be used repeatedly)
Note
If your filter is dynamic and hence can not be declared statically via these annotations,
use the
postprocess
callback instead.
Example
Let’s say, you only want to receive events that have a meta pair “priority”:“urgent” in their headers.
Here, you would use code like:
@Handler
@FilterByMeta(key="priority", value="urgent")
protected void apply(UserCreated created) {
// ...
}
This will add the additional filter defined by the @FilterByMeta
annotation to FactSpec
.
As a result, the filtering now takes place at the server side instead of
wasteful client side filtering (like in the body of the apply
method).
Only those Facts will be returned, that have a meta key-value-pair with a key of priority
and a value of urgent
.
@Handler
@FilterByMetaExists("priority")
protected void apply(UserCreated created) {
// ...
}
This will add the additional filter defined by the @FilterByMetaExists
annotation to FactSpec
.
Only those Facts will be returned, that have a meta key-value-pair with a key of priority
no matter what the value is.
5 - Optimistic locking
To make business decisions, you need a model to base those decisions on. In most cases, it is important that this
model is consistent with the facts published at the time of the decision and that the model is up-to-date.
For example, we want to ensure that the username is unique for the whole system.
In case of (potentially) distributed applications and especially in case of eventsourced applications,
this can be a difficult problem.
For sure what you want to avoid is pessimistic locking for all sorts of reasons,
which leaves us with optimistic locking as a choice.
On a general level, optimistic locking:
- tries to make a change and then to write that change or
- if something happens in the meantime that could invalidate this change,
discard the change and try again taking the new state into account.
Often this is done by adding a versionId
or timestamp
to a particular Entity/Aggregate to detect concurrent changes.
This process can be repeated until the change is either successful or definitively unsuccessful and needs to be
rejected.
For our example that would mean:
If a new user registers,
- check if the username is already taken
- if so, reject the registration
- if not, prepare a change that creates the user
- check if a new user was created in between, and
- repeat from the beginning if this is the case
- execute the change while making sure no other change can interfere.
In FactCast/Factus, there is no need to assign a versionId
or timestamp
to an aggregate or even have aggregates for
that matter.
All you have to do is to define a scope of an optimistic lock to check for concurrent changes in order to either
discard the prepared changes and try again, or to publish the prepared change if there was no interfering change in
between.
Let’s look at the example above:
Consider, you have a SnapshotProjection
UserNames
that we have seen before.
public class UserNames implements SnapshotProjection {
private final Map<UUID, String> existingNames = new HashMap<>();
@Handler
void apply(UserCreated created) {
existingNames.put(created.aggregateId(), created.userName());
}
@Handler
void apply(UserDeleted deleted, FactHeader header) {
existingNames.remove(deleted.aggregateId());
}
boolean contains(String name) {
return existingNames.values().contains(name);
}
// ...
In order to implement the use case above (enforcing unique usernames), what we can do is basically:
UserNames names=factus.fetch(UserNames.class);
if(names.contains(cmd.userName)){
// reject the change
} else {
UserCreated prepared=new UserCreated(cmd.userId,cmd.userName));
// publish the prepared UserCreated Event
}
Now in order to make sure that the code above is re-attempted until there was no interference relevant to the UserNames
Projection and also that the business decision (the simple if clause) is always based on the latest up-to-date data,
Factus offers a simple syntax:
/**
* optimistically 'locks' on a SnapshotProjection
*/
<P extends SnapshotProjection> Locked<P> withLockOn(@NonNull Class<P> snapshotClass);
Applied to our example that would be
UserRegistrationCommand cmd=... // details not important here
factus
.withLockOn(UserNames.class)
.retries(10) // optional call to limit the number of retries
.intervalMillis(50) // optional call to insert pause with the given number of milliseconds in between attempts
.attempt((names,tx)->{
if(names.contains(cmd.userName)){
tx.abort("The Username is already taken - please choose another one.");
} else {
tx.publish(new UserCreated(cmd.userId,cmd.userName));
}
});
As you can see here, the attempt call receives a BiConsumer that consumes
- your defined scope, updated to the latest changes in the Fact-stream
- a
RetryableTransaction
that you use to either publish to or abort.
Note that you can use either a SnapshotProjection
(including aggregates) as well as a ManagedProjection
to lock on.
A SubscribedProjection
however is not usable here, due to the fact that they are in nature eventual consistent,
which
breaks a necessary precondition for optimistic locking.
Also note that you should not (and cannot) publish to Factus directly when executing an attempt, as this would
potentially
break the purpose of the optimistic lock, and can lead to infinite loops.
In certain cases you might want to access the facts that were published inside the attempt block. Similar to the
org.factcast.factus.Factus#publish
method that has overloads where you can specify a Function<Fact, T> resultFn
,
you can pass a similar resultFn
or simply a Runnable
to the attempt
method. After successful publication this
function will be called with a List<Fact>
containing the published facts (in the order of publication). The return
value of the function will be returned by the attempt
method.
import java.time.Duration;
var passwordFactId = factus
.withLockOn(UserNames.class)
.attempt((names, tx) -> {
tx.publish(new UserCreated(cmd.userId, cmd.userName));
tx.publish(new UserPasswordChanged(cmd.userId, cmd.newPasswordHash));
}, facts -> {
// facts[0] -> UserCreated
// facts[1] -> UserPasswordChanged
// as published facts, both now have serial and fact id set.
// simple example only, do something more robust here
return facts.get(1).id();
});
// and now use the fact id as needed, e.g. in a waitFor
factus.waitFor(subscribedPasswordProjection, passwordFactId, Duration.ofSeconds(2));
For further details on failure handling,
please consult the JavaDocs, or look at the provided examples.
6 - Testing
Factcast comes with a module factcast-test
that includes a Junit5 extension that you can use to wipe the postgres database clean between integration tests.
The idea is, that in integration tests, you may want to start every test method with no preexisting events.
Assuming you use the excellent TestContainers library in order to create & manage a postgres database in integration tests, the extension will find it and wipe it clean.
In order to use the extension you either need to enable Junit-Extension-Autodetection, or use
@ExtendWith(FactCastExtension.class)
on your integration Test Class.
The easy way to get the full package is to just extend AbstractIntegrationTest:
public class MyIntegrationTest extends AbstractFactcastIntegrationTest { // ...
}
which gives you the factcast Docker image respective to the version of the dependency
you used from docker-hub running against a sufficiently current postgres, both being
started in a docker container (locally installed docker is a prerequisite of course).
If you want to be selective about the versions used, have a look at @FactcastTestConfig
which lets you pin the versions if necessary and allows for advanced configuration.
Also, in order to make sure, that FactCast-Server is NOT caching internally in memory,
you can add a property to switch it into integrationTestMode.
See Properties.
Local Redis
In case you are also using Redis, there is an additional factcast-test-redis
module.
When added as Maven dependency it is automatically picked up by the FactCastExtension
and starts a
local Redis instance.
7 - Handler Parameters
Inside projections, Factus uses methods annotated with @Handler
or @HandlerFor
to process events. These methods allow various parameters, also in combination, which can serve as “input” during event handling.
Common Handler Parameters
Parameter Type & Annotation | Description | valid on @Handler | valid on @HandlerFor |
---|
Fact | Provides access to all Fact details including header (JSON) and payload (JSON) | yes | yes |
FactHeader | the Fact header. Provides access to event namespace, type, version, meta entries and others | yes | yes |
UUID | the Fact ID of the Fact header | yes | yes |
FactStreamPosition | the FactStreamPosition that identifies the position of the given fact in the global fact stream | yes | yes |
@Nullable @Meta("foo") String | if present, the value of the fact-header’s meta object attribute “foo”, otherwise null | yes | yes |
@Meta("foo") Optional<String> | if present, the value of the fact-header’s meta object attribute “foo” wrapped in an Optional, otherwise Optional.empty | yes | yes |
? extends EventObject | an instance of a concrete class implementing EventObject . | yes | no |
Additional to these common parameters, Projections can add parameters to be used by handler methods.
For instance handler methods on @RedisTransactional projections that should use:
Examples
@Handler
Here are some examples:
// handle the "SomeThingStarted" event.
// deserialization happened automatically
@Handler
void apply(SomethingStarted event) {
var someValue = event.getSomeProperty();
...
}
// handle the "SomethingChanged" event.
// additionally use information from the Fact header
@Handler
void apply(SomethingChanged event, FactHeader header) {
int eventVersion = header.version();
String someMetaDataValue = header.meta().get("some-metadata-key");
...
}
// use multiple parameters
@Handler
void apply(SomethingReactivated event,
FactHeader factHeader,
UUID factId,
Fact fact) {
...
}
These examples were all based on handling events which
The next section introduces a more direct alternative.
@HandlerFor
The @HandlerFor
annotation allows only direct access to the Fact data like header or payload without any deserialization.
// handle "SomethingAdded" events in their version 1
// living in the "test" namespace
@HandlerFor(ns = "test", type = "SomethingAdded", version = 1)
void applySomethingAdded(Fact fact) {
String payload = fact.jsonPayload();
...
}
// also here, multiple parameters can be used
@HandlerFor(ns = "test", type = "SomethingRemoved", version = 2)
void applySomethingRemoved(FactHeader factHeader, UUID factId, Fact fact) {
...
}
Full Example
See here for the full example.
8 - Metrics
Like the FactCast server, also Factus makes use
of micrometer.io metrics.
Metric namespaces and their organization
At the time of writing, there are three namespaces exposed:
factus.timings
factus.counts
factus.gauges
Depending on your micrometer binding, you may see a slightly different spelling in your data (like '
factus_timings`, if your datasource has a special meaning for the ‘.’-character)
The metrics are automatically tagged with
- the emitting class (
class
tag) - the name of the metric (
name
tag)
Existing Metrics
At the time of writing (Factcast version 0.3.13) the following metrics are supported:
Counted
transaction_attempts
- how often was a transaction retried. See Optimistic Locking for more backgroundtransaction_abort
- how often was an attempted transaction aborted
Gauged
Timed
managed_projection_update_duration
- duration in milliseconds a Managed Projection took to updatefetch_duration
- duration in milliseconds it took to fetch a Snapshot projectionfind_duration
- duration in milliseconds it took to find a specific Aggregateevent_processing_latency
- for those facts that arrive after catchup: time difference in milliseconds between a fact was published and recieved by a client. (In case of batch processing, this is only reported for the first/oldest fact of a batch)
9 - Tips
This section contains some tips and tricks that you might find useful to improve performances or to cover some corner use cases.
@SuppressFactusWarnings
Similar to java.lang.SuppressWarnings
, you can use this annotation to suppress warnings. You could notice these when factus encounters a class violating good practices (for instance when scanning your projection) the first time.
The annotation can be scoped to a type, method or field declaration.
It requires a value, which specifies the type of warning(s) to suppress. At the time of writing (Factcast version 0.5.2), the allowed values are:
SuppressFactusWarnings.Warning.ALL
suppresses all Factus related warningsSuppressFactusWarnings.Warning.PUBLIC_HANDLER_METHOD
suppresses “Handler methods should not be public” type of warning, caused by projection handler
methods having a public scope